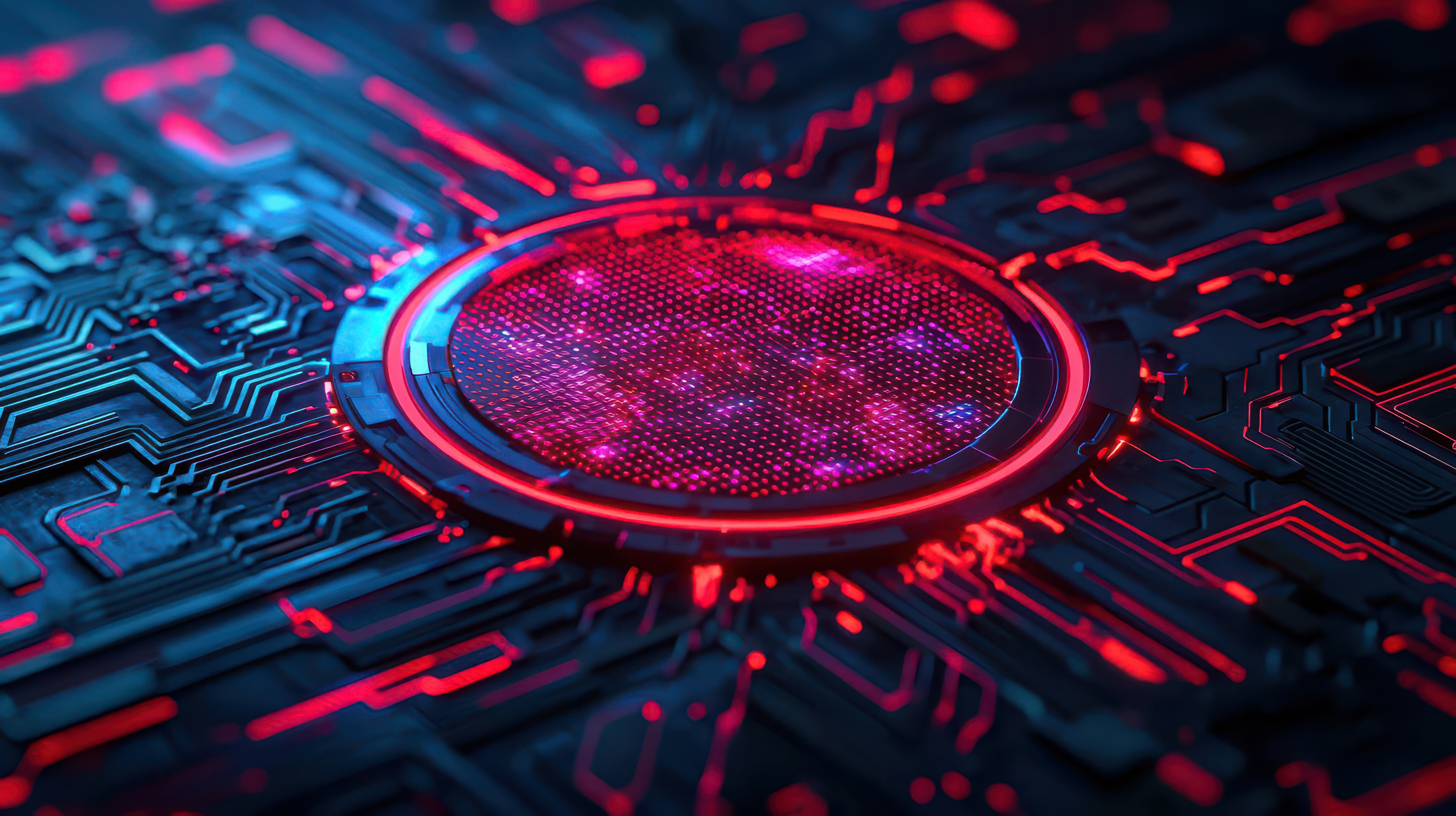
Right now, we’re in the middle of an AI boom. Healthcare, agriculture, e-commerce, software development, manufacturing – groundbreaking advancements in AI are transforming industries on a scale that we could not have predicted only a few decades ago.
Meanwhile, the development of a viable nuclear fusion reactor presents a range of engineering challenges. Given the progress fuelled by AI across many sectors, it is no surprise that fusion scientists are turning to AI to innovate and optimise reactor designs in an effort to accelerate fusion research.
At COP29, the Clean Air Task Force launched its report exploring how AI is being used to develop nuclear fusion in areas such as advanced diagnostics, high-temperature superconductors (HTS), materials selection, inertial fusion energy, and tritium breeding. Here, we explore some of the areas where AI could be a gamechanger for the fusion industry.
A fundamental task for all fusion reactor types is to confine a plasma at a sufficient density and temperature long enough to achieve a self-sustaining nuclear fusion reaction. The Swiss Plasma Center in Lausanne operates a variable configuration tokamak having a specialized plasma shaping capability for producing diverse plasma shapes without requiring hardware modifications. In 2022, Google’s UK-based AI company DeepMind worked with the Swiss Plasma Center to develop a single neural network capable of automatically controlling the 19 separate magnetic coils of the tokamak.
The popular tokamak approach suffers from many dynamic instabilities of the plasma that can result in loss of confinement. In 2024, researchers at the DIII-D National Fusion Facility demonstrated a method for instability prevention using deep reinforcement learning to predict the occurrence of “tearing” instabilities based on real-time observations of a tokamak plasma profile. Rather than using a traditional pre-programmed operation, the method uses an AI-controller to adaptively control actuators based on the model’s predictions in tens of milliseconds to prevent the instabilities from occurring.
Many large-scale fusion machines demand the scaling up of HTS magnet production. However, it is essential that material quality is maintained. AI techniques are now being used to predict defects and optimize manufacturing parameters to improve manufacturing efficiency and reduce costs.
AI can even be used to find new materials. Researchers from the U.S. Department of Energy created an AI model which has successfully identified new alloys for shielding components in a nuclear fusion reactor. Effectively, they could bypass long periods of trial and error to find candidate materials.
An ongoing project has been proposed in Europe to create an AI-driven database of materials suitable for fusion reactors. Machine Learning will be used to help manage and analyse experimental data to predict material properties. Although intended primarily to support the ITER and DEMO projects, the database will be public and will undoubtedly become a valuable resource across the sector.
The University of Manchester are collaborating with the UK Atomic Energy Authority (UKAEA) to build a digital twin of a fusion reactor using the NVIDIA Omniverse simulation platform. Considerable costs can be saved by first testing the digital twin to explore hypothetical scenarios, before deploying changes to the physical system. The digital twin will also enable the whole reactor design to be visualised rather than just individual components.
Tokamak Energy have already developed their own AI-powered digital twin computer which can simulate multiple experiments at the same time based on their high-field compact spherical tokamak design. They have said AI has the potential to knock years off timelines and cut costs.
AI is also being used in a variety of ways to advance ICF, including optimizing target design to improve structural symmetry with the goal of increasing energy gain. Generative AI is also being used to improve laser precision for irradiating the fuel target.
There is a huge demand for sustainable energy sources to power AI-data centres. The CEO of OpenAI is a lead investor in US nuclear fusion company Helion Energy who have raised an impressive $500 million to date. Last year, he said that an energy “breakthrough” will be necessary to support the growth of AI. The future of AI’s infrastructure might very well depend on the success of fusion energy.
In January 2025, the UK government announced that it will deliver the first AI Growth Zone at the headquarters of the UKAEA. This initiative aims not only to invest in the UK’s AI market but also to boost fusion energy research. Are we likely to see other governments follow suit?
It seems inevitable we will continue to see this convergence of AI and fusion energy research in both public and private sectors. Ultimately, nuclear fusion could be the perfect solution to meet the exceptional power demands of our growing AI infrastructures. Until then, the progress of AI will continue to benefit the pursuit of commercial fusion energy.
If we are to overcome the challenges of nuclear fusion, the implementation of AI itself will play an essential role.
Harry is a patent attorney in our engineering and ICT team. Harry's areas of expertise include Power generation, Nuclear reactor safety systems, Image processing, Radar systems, Optical systems, Cryptography Medical devices including wearables, laryngoscopes and surgical endoscopes.
Email: harry.westhead@mewburn.com
Our IP specialists work at all stage of the IP life cycle and provide strategic advice about patent, trade mark and registered designs, as well as any IP-related disputes and legal and commercial requirements.
Our peopleWe have an easily-accessible office in central London, as well as a number of regional offices throughout the UK and an office in Munich, Germany. We’d love to hear from you, so please get in touch.
Get in touch