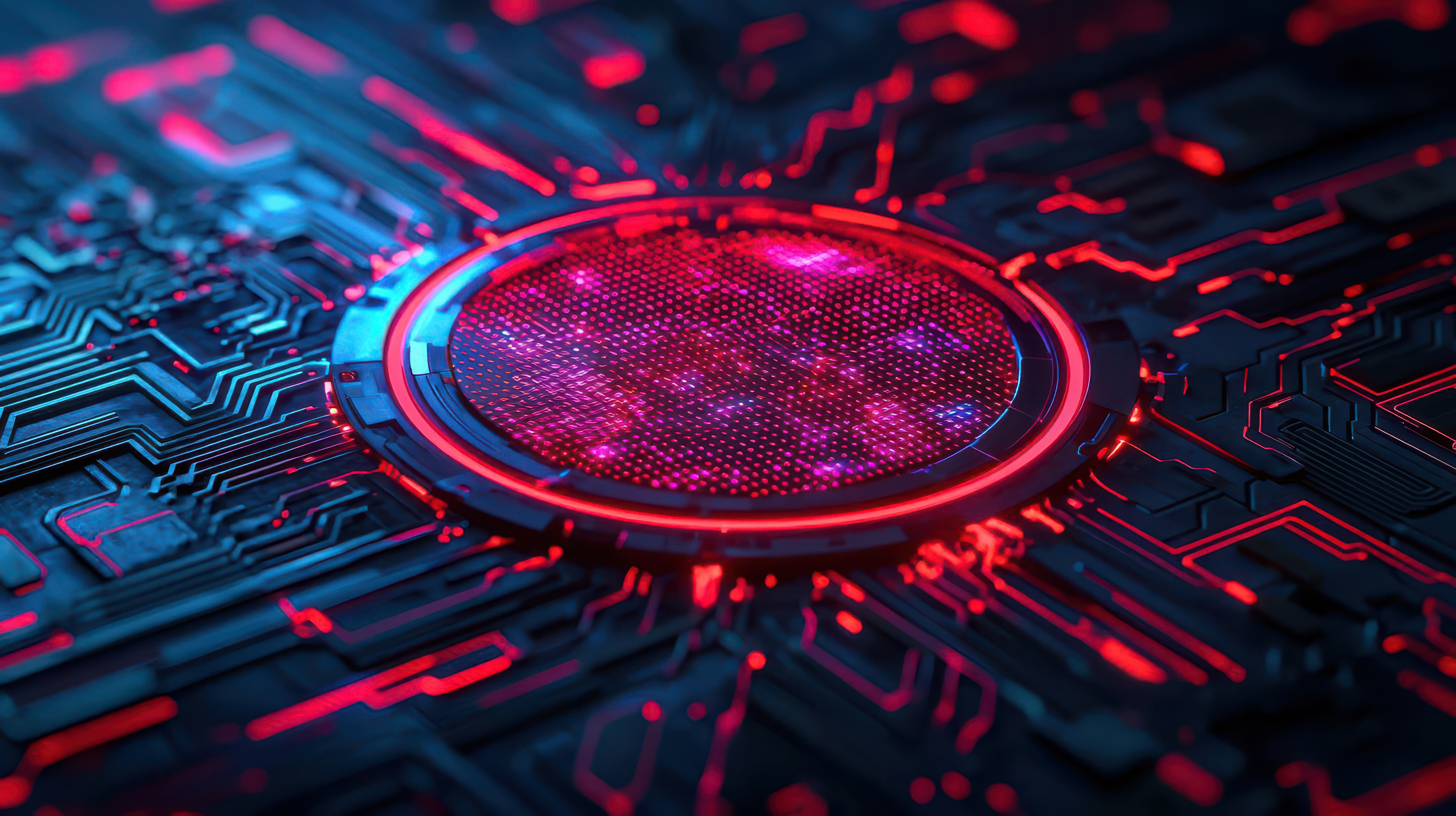
AI tools are increasingly being used in healthcare settings, with applications ranging from analysing brain scans to identifying people who would benefit from extra support from their care providers. While these tools are incredibly powerful, there is concern that bias in their algorithms could negatively affect patient outcomes. AI bias can be defined as AI that makes decisions that are systematically unfair to certain groups of people. This is an issue that every industry using AI will have to address, but in a sector as critical as healthcare, the need to limit AI bias to avoid exacerbating existing inequalities is especially important.
The issue of bias in healthcare is of course not limited to AI; the AI algorithms are simply reflecting existing biases. For example, the Framingham Risk Score has been widely used to predict cardiovascular risk for over 20 years. Hurley et al. found that this risk score showed variable strength of association between the risk score and cardiovascular disease mortality for different ethnic groups. This is partly due to the predominantly white cohorts used to develop it. Women are also more likely to have missed and delayed cardiovascular disease diagnoses compared to men, despite it being among the leading causes of female mortality. Females have been underrepresented in clinical trials in cardiology, likely contributing to the poorer health outcomes seen for women with cardiovascular disease.
There will always be some bias in the data used, and so there will always be some bias in the tools developed, whether they are AI-based or not. The introduction of AI tools that are validated to limit bias to an acceptable level gives the opportunity to reduce the variation in patient outcomes between different groups that is seen today.
There are many causes of bias in AI tools. A major one is there being a disproportionate sample size for a particular group of people in the training data. For example, a 2016 study found that in the field of genomics, 80% of collected data was from Caucasians. If there are more samples from a particular group in the training data, it follows that any AI tool is going to give more accurate predictions for that given group.
Another cause may be that the parameters selected as inputs to a model reflect existing biases against certain groups. A 2019 study found that a widely used AI tool that screened patients for high-risk care management programs was racially biased. The tool assigned each patient a risk score to determine how much they would benefit from being included in a high-risk care management program, with a higher score indicating that being part of the program would be more beneficial. Despite the algorithm specifically excluding race from contributing to the score, it was found that black patients had significantly more active chronic conditions at a given risk score than white patients. In other words, black patients were significantly more ill than white patients who had the same risk score. This was because the algorithm was predicting the future cost of care as a measure of each patient’s health. Historically, the black patients had generated less medical expense due to a variety of socioeconomic factors and so had to be comparatively more ill to receive the same risk score in order to have the same chance of being recommended for the program. This shows that simply excluding protected characteristics from algorithm input parameters is not enough to avoid AI bias.
Another issue that arises is the opaque nature of many AI algorithms, particularly those which use deep learning, a subset of machine learning which imitates the behaviour of the human brain to learn from large amounts of data. This makes it very difficult to extract how a model has come to a decision. Transparent and explainable AI has received some interest, however deep learning has already been used for a wide variety of tasks, including cancer diagnosis and DNA sequencing. It therefore seems unlikely that the use of deep learning techniques in healthcare will be restricted.
There are several ways in which AI bias can be reduced or mitigated. One of the most straightforward is so called human-in-the-loop system, where a human considers the information given by an AI tool in the wider context of an issue before taking a final decision, rather than an AI tool taking a final decision with no human input. For example, in the case discussed above where the AI algorithm was found to be racially biased, a clinician took the risk score calculated by the AI algorithm, while considering other information in each patient’s medical record, to decide whether or not to recommend a patient to a high-risk care management program, rather than the AI algorithm simply deciding which patients would be recommended to a high-risk care management program. This type of human-in-the-loop system limits the damage a potentially biased AI tool could cause.
Another technique is to adjust the algorithm itself. Mittermaier et al. reduced the bias in a technical sense of an AI algorithm which was used to assess surgeon skill from video, by training a separate model to assess the relevance of each video frame. This enabled the surgeon-skill prediction model to discard frames which may previously have caused inaccuracies. This reduced the model’s bias against surgeons who had lower quality videos used to assess their skill level.
A long-term strategy to mitigate AI bias is to ensure that the teams developing the AI models receive appropriate diversity training and include people from a range of both social and technical backgrounds, for example to include clinicians, who understand the context in which the models will be used. This would help these teams to recognise and mitigate against bias at every stage of development, from the collection and cleaning of data to the deployment of the AI tool. This would also ensure that tools for a range specific populations are developed, the advantages of which can already be seen with AI-powered apps such as Midday, which is used to support women through the menopause and b-rayz, which aims to reduce the number of missed early stage breast cancers.
A final important point to note is that there will always be some bias in an AI model, the same as each person will always have some level of unconscious bias. Therefore, an important question is what level of bias is acceptable, which is analogous to the accuracy threshold a model must achieve. This question is as yet unanswered. From a regulatory standpoint, the STANDING Together initiative, which is led by researchers at the University Hospitals Birmingham NHS Foundation Trust and the University of Birmingham, released recommendations in October 2023 which aim to “tackle inequitable performance of AI health technologies”. These provide a framework for high-quality dataset documentation and provide recommendations for the selection and use of health datasets, including identifying groups who may be at risk of disparate performance or harm from the AI tool, evaluating the performance of the AI tool for these identified groups, justifying the datasets used in the context of the intended use population, and reporting methods used to intentionally modify performance across groups.
With the inevitable increase in the prevalence of AI technologies in healthcare, the importance of reducing and mitigating AI bias will become increasingly important. These technologies have the potential to improve patient outcomes and decrease the inequalities seen in healthcare today. It will be interesting to see how AI bias is mitigated against while new and exciting AI-based healthcare technologies are developed.
Joe is a trainee patent attorney in the Engineering practice group, working in the Bristol office. Joe graduated from the University of Bath with an integrated Master’s degree in Mechanical Engineering (Meng). He was part of the team which launched Team Bath Heart, a student-run competition team, aimed at designing a total artificial heart from scratch. As part of the team he focused on the human-artificial heart interface.
Email: joe.egelstaff@mewburn.com
Our IP specialists work at all stage of the IP life cycle and provide strategic advice about patent, trade mark and registered designs, as well as any IP-related disputes and legal and commercial requirements.
Our peopleWe have an easily-accessible office in central London, as well as a number of regional offices throughout the UK and an office in Munich, Germany. We’d love to hear from you, so please get in touch.
Get in touch