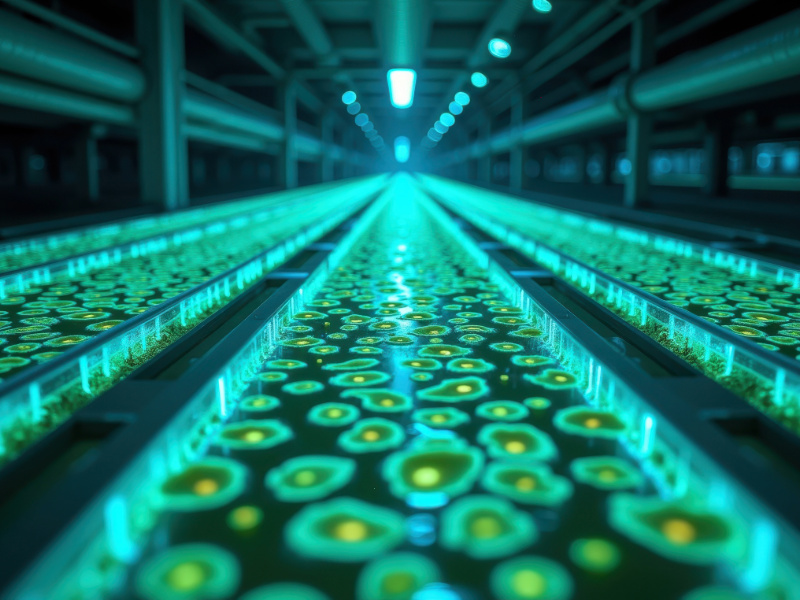
Putting “AI” in the title of your paper, or indeed in the name of your company, seems to have become a sure way to get traction in many fields. Bioinformatics, and in particular medical informatics is no exception.
The past few years have seen crucial advances in the field of automated image analysis, leading to a flurry of applications in many fields. Testimony to the growing importance of image analysis in the field of bioinformatics is the fact that the EMBL-European Bioinformatics Institute (one of the main providers of open data resources for the worldwide scientific community) has recently launched a database called BioImage Archive that “stores and distributes biological images that are useful to life-science researchers”. In short, images are the newest addition to the treasure-trove of data that is available for bioinformatics researchers to draw on to learn about biology.From a medical application point of view, current methods for image analysis are so good at picking up image features associated with diseases that they have led to popular press headlines indicating that AI could match or even outperform doctors in the near future. But is this true, what is this all about anyway, and where is this all going?
Most of what is behind the current AI buzz could also be called machine learning, and in particular deep learning, applied to image analysis. Deep learning refers to a class of machine learning algorithms that use models based on artificial neural networks with multiple layers that progressively extract higher level features from a raw input. For example, applied to image analysis, the first few layers may identify edges while the higher layers may identify complex shapes or patterns by combining lower level features according to learned patterns of “importance”. Convolutional neural networks (CNNs) are the most popular type of deep neural networks used in image analysis, because they currently perform best at the task. CNNs are inspired by the organisation of the animal visual cortex in that nodes (cortical neurons) in one layer respond to signals (stimuli) from a restricted set of nodes (region of the visual field known as the receptive field) in the previous layer, where receptive fields partially overlap to cover the entire field.
CNNs have been known for a few years but their popularity exploded when a deep CNN achieved an unprecedented classification accuracy at the 2012 ImageNet Large Scale Visual Recognition Challenge (ILSVRC – a competition where research teams get to evaluate their algorithms on the data from the ImageNet database in terms for accuracy on several visual recognition tasks). In 2015, another deep CNN algorithm outperformed humans on specific visual recognition tasks, which brought deep learning into the headlines.
Since then, algorithms of this type have been applied to perform image and video recognition (computer vision) and image classification in many fields from facial recognition to driverless cars, medical imaging, etc. Indeed, these methods are more or less agnostic to the type of image or the type of information that one is trying to glean from the images. After all, an image is an image, and features in an image are patterns of pixels. As such, they are able to “learn” to recognise patterns in images of pretty much any kind, and associate these patterns with human-defined concepts such as “what is the object shown on this image”, “who are the people shown on this image” or “does this image contain something that looks like a tumour”?
In the field of medical imaging, the potential for these technologies is unprecedented. Partially this is because when the task is well defined, these algorithms do perform very well as identifying what they have been taught to identify. Partially this is because they do so much quicker than humans can – and that would still be true even if health systems were much better staffed than they are. Potential clinical applications range from the early diagnosis of macular degeneration from routine eye scans, to the identification of embryos with the best chance of implantation in IVF, via early diagnosis of various cancers such as breast cancer from mammography data.
Well, yes and no. The algorithms mentioned above were only shown to outperform humans on limited tasks, such as recognising objects as belonging to one of about a thousand categories. For these tasks, these algorithms are indeed very good. For specific medical tasks such as the classification of dermoscopic melanoma images, rigorous studies have shown that CNNs could outperform doctors. However, machine learning algorithms are trained to recognise a limited number of things that they have seen before. Of course that limit is ever increasing, but a human brain can recognise a much larger number of things, and crucially can interpret the context of an image and draw some inference about e.g. the future based on this. For example, a human can see an image with a pedestrian and a car, and not only recognise these things as “people” and “car”, but also predict that the driver looks inebriated, that the pedestrian looks distracted, and that this is a dangerous situation for the pedestrian to be in. A machine learning algorithm that has not been trained to find signs of inebriation or distraction, or to determine the heading direction of the car simply won’t be able to put 2 and 2 together to make that prediction.
Having said that, such approaches are particularly well suited to look for:
As such, their potential to improve healthcare by assisting doctors in pointing out to things they may not have seen, and alerting them to conditions that may not be visible to them, is tremendous.
Further, these algorithms may bring previously unavailable “artificial brain power” to patients that simply may not have access to (human) doctors. For example, these technologies may be able to reach patients in remote areas, areas that are dangerous for doctors to access (because of war, epidemics, etc.) or areas that simply do not have enough doctors to keep up with the demand.
One thing is for sure, it will include AI. To which extent it does so, and to which extent this is visible to patients will depend on the application, the health system considered etc. Most likely, this AI will in the near future be another tool that is routinely available to doctors, and embedded in their usual practice in the form of e.g. augmented imaging systems that not only show an image but display a conclusion from it and/or point out to parts of the image worth a closer look.
Following up on and interpreting insights from the AI will likely remain the task of a doctor, who knows the patient, can interpret the wider medical context of the image, and ultimately can translate the insight into an appropriate course of action. Data integration (the combination of information from multiple sources including e.g. medical records, genomic data, etc.) has the potential to enable AI to make progress into this territory as well. However, with the great power of data integration comes great responsibility (in terms of at least privacy and medical liability). Many ethical and legal issues will need to be resolved for this to safely become a reality, without eroding public trust. In the longer term, as tech optimists we believe that AI will match human performance in an increasing number of ways, and this will slowly but surely change how much we are able to trust a machine’s judgment.
Camille is a Partner and Patent Attorney at Mewburn Ellis. She does patent work in the life sciences sector, with a particular focus on bioinformatics/computational biology, precision medicine, medical devices and bioengineering. Camille has a PhD from the University of Cambridge and the EMBL-European Bioinformatics Institute. Her PhD research focused on the combined analysis of various sources of high-content data to reverse engineer healthy and diseased cellular signalling networks, and the effects of drugs on these networks. Prior to that, she completed a Master’s degree in Bioengineering at the University of Brussels and a Masters in Computational Biology at the University of Cambridge.
Email: camille.terfve@mewburn.com
Our IP specialists work at all stage of the IP life cycle and provide strategic advice about patent, trade mark and registered designs, as well as any IP-related disputes and legal and commercial requirements.
Our peopleWe have an easily-accessible office in central London, as well as a number of regional offices throughout the UK and an office in Munich, Germany. We’d love to hear from you, so please get in touch.
Get in touch