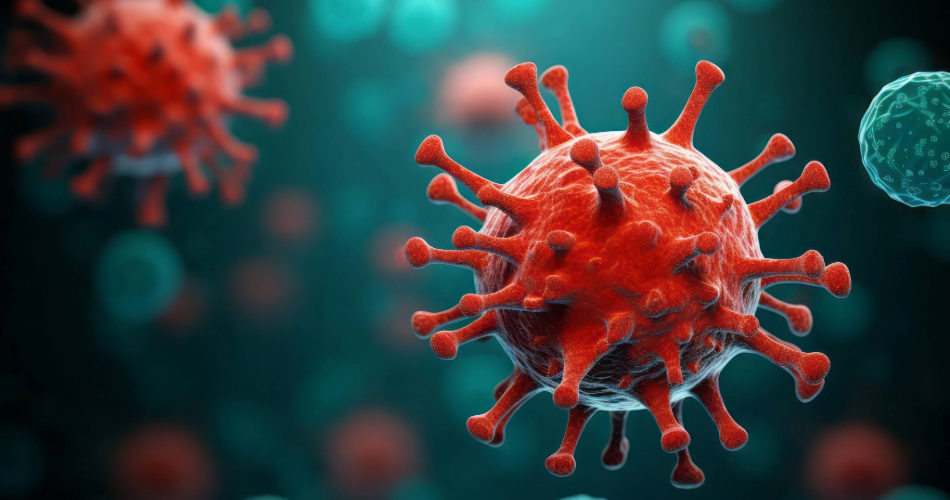
During the announcement of the first survey of the entire human genome by the Human Genome Project, Dr Francis Collins commented that “many tasks lie ahead if we are to learn how to speak the language of the genome fluently”.
The application of generative AI may be moving us closer to that goal. Generative AI is artificial intelligence capable of creating new content. A ubiquitous example is ChatGPT, a large language model capable of generating new text in response to a prompt. A new generation of biotech startups are now seeking to apply this technology to the “language of the genome” – nucleotide sequences.
Viruses can have either RNA or DNA based genomes, in single strand or double stranded form. They can mutate quickly, meaning our immune system no longer recognises them (hence a yearly flu jab, rather than a single vaccine protecting you over your lifetime). We can now predict the mutations that will allow viruses to become unrecognisable (termed immune escape) using AI language models.
Language models are created by learning the probability of a ‘token’ (such as an English word) appearing in its ‘sequence context’ (such as a sentence). Here, instead of words the tokens are short nucleotide sequences, within their genomic context. The researchers focussed on two read-outs of the model, “grammaticality” and “semantics”. “Grammaticality” refers to how well a token fits the “rules” of a language (i.e., is it grammatically correct?)
For example, the word “blue” in “The quick blue fox jumps over the lazy dog” would have high grammaticality, even if we think it’s a bit of an odd sentence. Semantics describes the meaning of the token, i.e. a switch from “blue” to “brown” would constitute a semantic change, but no significant change in grammaticality.
Applying these two components to viral sequences can provide some unique conclusions. Researchers predicted that grammaticality would capture viral fitness (if your viral genome obeys the “rules” of viral genomes, you will probably be able to propagate), while semantic change would correspond to a change in protein function and antigenic escape (if you change the “meaning” of your gene, you’ll end up with a protein with a new function that the human immune system probably won’t recognise).
Despite being trained on no functional or structural data, a language model trained on viral genomes was able to predict viral mutations that would lead to immune escape (requiring high grammaticality accompanied by semantic change). This led to insights such as showing that the sequence encoding the SARS_CoV_2 Spike protein, the large transmembrane protein that gives COVID its “spiky” appearance, has high “escape potential” within both the receptor-binding domain and the N-terminal domain.
Such insights could be helpful in future vaccine design by helping to predict if a viral particle is likely to evade our immune system, and where to target our vaccine efforts. A site with enriched “escape potential” has experienced functional mutations compared to known viral sequences. Targeting low “escape potential” regions are likely to be correlated with greater evolutionary conservation, and therefore present better targets.
How about using these models to create new proteins based off new nucleotide sequences?
ProGen, a ‘conditional’ language model developed by Salesforce Research, can generate nucleotide sequences that encode functional proteins. A conditional language model can be prompted to generate language with specific properties after learning from a data set tagged with these properties. For example, if you train a language model on a variety of English text, it can generate convincing new written text in English. However, you can also tag certain sentences (e.g. these ones are written in a formal style, and these ones are informal). The model will then be able to generate text specifically written in an informal style when prompted.
By training their model on 280 million protein sequences tagged by protein family (of which there were around 19,000), ProGen was able to generate artificial lysozyme protein sequences when prompted with 5 distinct lysozyme families. These artificial enzymes had similar catalytic efficiencies as their natural counterparts, but with sequence identity as low as 31.4%. Despite this low identity, the artificial protein structure was highly similar to their natural counterparts.
This low sequence identity is particularly exciting – because it implies that AI models can construct a sequence for an enzyme active site from ‘first principles’, rather than modifying existing sequences. This would differentiate generative AI from traditional methods to generate new enzymes that involve mutating existing sequences and observing the resulting functional changes. Some companies are already putting this technology into effect.
Biomatter, a Lithuanian start up, uses generative AI to both modify existing enzymes and generate new ones. Back in 2019, they published a paper in Nature Machine Intelligence which set out their success in creating the first enzymes using generative AI. Biomatter’s Intelligent ArchitectureTM combines generative AI and physics models. Importantly, it promises to keep experimental validation to a minimum, allowing for enzyme design to proceed quickly in a dry lab setting. Traditionally, enzyme design can involve multiple rounds of performance testing followed by generating additional mutants. By involving generative AI in this process, enzyme development timelines can be shortened from years to a few months. They recently raised €6.5 million in a seed funding round, setting them up for future innovation.
Other companies want to reduce the potential downsides of traditional wet lab elements even further. Imperagen, a University of Manchester spin-out, aims to combine generative AI and laboratory automation to design and manufacture new enzymes. By fully automating the wet lab validation aspect of enzyme development, they are seeking to shave even more time off the development pipeline.
So where do patents come into all this?
There are certainly exciting opportunities to patent new enzymes. Patent applications for newly developed enzymes can have their scope narrowed by prior art disclosing sequences with high identity. This would not be the case for a new enzyme with very low homology to its natural counterparts. As always, providing sufficient data to show that functionality is maintained over a group of different sequences would be very helpful to support a broader scope.
There are also opportunities to protect the platforms themselves. A host of OpenAI patent applications covering the use of their large language models were recently published, demonstrating a shift in strategy from protecting their technology through trade secrets rather than patents. Methods using AI for novel peptide sequence generation certainly have potential for protection as well.
Isobel is a trainee patent attorney in the life sciences team. She has a BSc in Biochemistry from Imperial College London. Her final year project involved comparing the genomic response of human and rats post burn to help understand the evolution of the burn response in humans.
Email: isobel.fisher@mewburn.com
Our IP specialists work at all stage of the IP life cycle and provide strategic advice about patent, trade mark and registered designs, as well as any IP-related disputes and legal and commercial requirements.
Our peopleWe have an easily-accessible office in central London, as well as a number of regional offices throughout the UK and an office in Munich, Germany. We’d love to hear from you, so please get in touch.
Get in touch