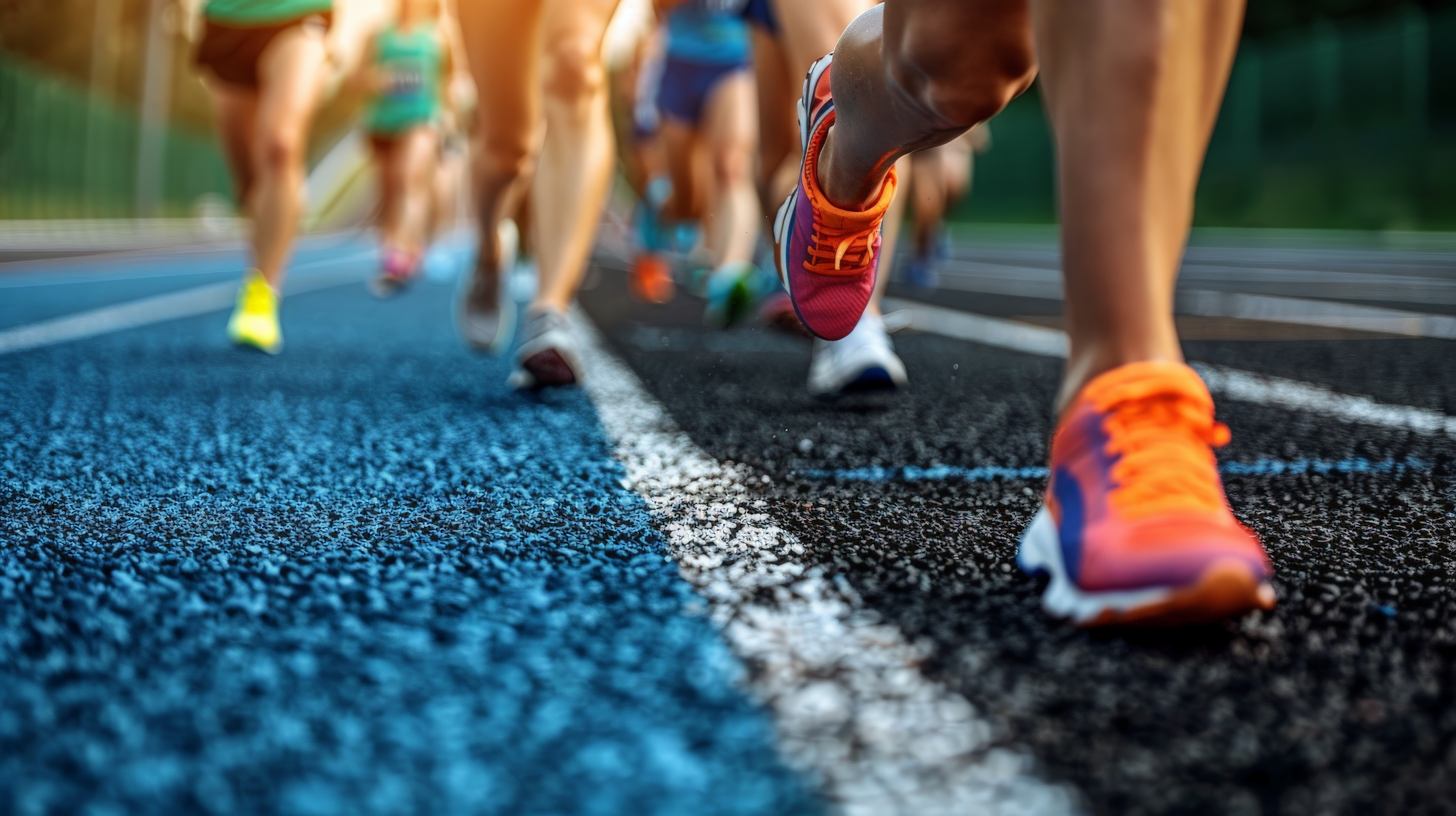
Alchemab’s artificial intelligence platform enables the identification of antibodies for some of the toughest jobs in pharma, from treating Alzheimer’s to beating cancer.
Forward: features are independent pieces written for Mewburn Ellis discussing and celebrating the best of innovation and exploration from the scientific and entrepreneurial worlds.
Drug companies traditionally focus on people who are ill – they look at what’s gone wrong and how to put it right. But Alchemab Therapeutics is doing the opposite.
Alchemab studies people who are well, those who have overcome cancer, say, or resisted Alzheimer’s – to find out what has gone right.
The premise is that these resilient individuals have antibodies that have allowed them to beat or get the better of disease, and if these antibodies can be identified, they could be used to develop new drugs for everyone else.
‘There is really nice evidence that people can naturally produce these autoantibodies that can protect them against diseases such as cancer and neurodegeneration, and that’s what we’re looking for,’ says Dr Jake Galson, Alchemab’s vice-president of technology.
‘But the interesting thing about antibodies, and what makes this really hard to do, is that antibodies are incredibly diverse. One individual can produce billions and billions and billions of different variants and we have to pinpoint the protective antibody for the disease of interest.’
To do that, Alchemab uses AI to find antibodies that are shared by people who have resisted or recovered from a disease, but are not found in those who have succumbed to the particular illness.
Dr Jake Galson, Vice-President of Technology, Alchemab
The reason that works, says Galson, ‘is because the composition of antibodies that any one individual has is largely unique to them, so there’s actually quite a small overlap between individuals. Those antibodies that do overlap are likely related to a common disease stimulus’.
Rather than looking for antibodies with the same, or similar, DNA sequence, the drug discovery engine searches for antibodies that are performing a similar role, regardless of their make-up.
‘We know that antibodies can have quite different sequences but similar functions,’ says Galson. ‘That can allow you to pull out many more of those common antibodies than you would find using traditional methods.’
Once an antibody has been identified, the next step is to find its target.
‘We use experimental methods where we can display a large number of human proteins and try to understand which one of these the antibody is binding to,’ says Galson. ‘Again, that’s the reverse of the traditional drug discovery approach.
‘In traditional drug discovery, you have the disease and the drug targets that are relevant for that, and then you develop an antibody that binds to one of those targets.
‘Our approach allows us to find drug targets that other people haven’t thought about before.’
Antibodies have conventionally been discovered using time-consuming techniques that involve immunising animals or genetically engineering phages – viruses that infect bacteria.
AI offers the promise of speeding things up and cutting costs, so it is increasingly being used in antibody design and development, albeit mainly by companies that are following the well-trodden path of looking at what’s gone wrong (identifying a drug target) and then trying to put it right (by developing an antibody).
What does Galson think are the most exciting applications of the technology, Alchemab’s aside?
‘What’s really exciting and what some companies are trying to do is to say: “Here’s a drug target, use AI to generate me the best possible antibody or set of antibodies against that target really, really quickly and really effectively,”’ he says.
‘But we don’t as yet have “anywhere near” the amount of training data needed to make sense of antibodies’ incredible diversity and generate de novo antibody sequences, he adds.
With enough data, it may be possible to create antibodies for hard-to-crack targets, says Galson. These include G-protein-coupled receptors – proteins that have been implicated in a range of diseases, from diabetes and depression to Alzheimer’s and cancer.
What is more tractable, says Galson, is using AI to optimise antibody development by, for example, selecting the most promising antibodies from a pool of candidates or tweaking existing antibodies to create more effective versions.
Another challenge is that the way antibodies bind to their targets is something of a mystery.
Unlike other proteins, antibodies bind using floppy loop-like structures and these have proved particularly difficult to model with AI.
‘The easiest way to think about antibodies is [that they’re] like hands,’ says a colleague of Dr Galson’s. ‘If you look at our hands, when you grab on to different things your fingers form different conformations but your palm remains relatively static – and that’s a really good analogy for what antibodies are like.
‘Most of an antibody is quite easy to model, but there are these floppy bits, like our fingers, and it is hard to work out what they’ll do when they grab on to different objects.
‘So you have this weird dynamic, where the most important part of your molecule is the thing that is most difficult to model and work out. It’s quite different to other protein-protein interfaces.’
Training data is also viewed as being so valuable that most of it is proprietary.
Even when the data used to train an algorithm came from a public dataset originally, it may have been filtered or restructured in a way that gives a company an edge – and that reworked dataset is unlikely to be released.
‘They’ll tell you about the modifications they made, they’ll maybe tell you the logic, but the actual dataset itself won’t be released,’ says Dr Galson’s colleague. ‘And I think it has been holding the field back, especially earlier on.
‘Circa 2022, 2023, it felt like every other academic group and pharmaceutical company was making a new large language model for antibodies, using very similar model architectures, using very similar training regimens, but everyone had their own way of filtering data.’
Galson adds: ‘Often the companies that have the best data don’t have the machine learning innovation to do anything with that data. Or they are big pharmas that have a lot of historic data that hasn’t been stored in a way that makes it easy to retrieve and use.
‘There’s a bit of a disconnect with a lot of the innovation happening within startup companies that don’t actually have particularly good datasets and not so much innovation happening within the companies that do have good datasets.’
There are examples, though, of big pharma working closely with – and in some cases acquiring – companies with expertise in machine learning.
A more open data culture would help the field advance as a whole, say the team at Alchemab, as would more crosstalk between computational scientists and chemists.
Each has skills that are of value to the other and so a ‘culture that allows the open exchange of ideas and information would be hugely beneficial’.
There is also a lot of hype, and even slightly wishful thinking, that needs to be cut through too, says Galson, if the amazing ideas of today are to bear fruit in ten years’ time.
The potential is, however, huge.
‘What we really want is a general-purpose machine learning model that can understand antibody-target interactions and find promising antibody drugs for any given target,’ says Galson.
‘The technology isn’t there yet, but it is improving all the time and we’ll get there. I know we will.’
Camille Terfve, Partner and Patent Attorney at Mewburn Ellis, comments:
"The complexity and potential functionalities of the immune system is such a vast, mostly unexplored space that we need all the help we can get to explore it productively. AI is a natural fit for this, and it is so exciting to see highly innovative antibody companies like Alchemab making the most of combined antibody and AI expertise. From an IP perspective this hybrid, very fast paced field also presents unique challenges and opportunities that it is our privilege to deal with every day.”
Written by Fiona MacRae
Camille is a Partner and Patent Attorney at Mewburn Ellis. She does patent work in the life sciences sector, with a particular focus on bioinformatics/computational biology, precision medicine, medical devices and bioengineering. Camille has a PhD from the University of Cambridge and the EMBL-European Bioinformatics Institute. Her PhD research focused on the combined analysis of various sources of high-content data to reverse engineer healthy and diseased cellular signalling networks, and the effects of drugs on these networks. Prior to that, she completed a Master’s degree in Bioengineering at the University of Brussels and a Masters in Computational Biology at the University of Cambridge.
Email: camille.terfve@mewburn.com
Our IP specialists work at all stage of the IP life cycle and provide strategic advice about patent, trade mark and registered designs, as well as any IP-related disputes and legal and commercial requirements.
Our peopleWe have an easily-accessible office in central London, as well as a number of regional offices throughout the UK and an office in Munich, Germany. We’d love to hear from you, so please get in touch.
Get in touch