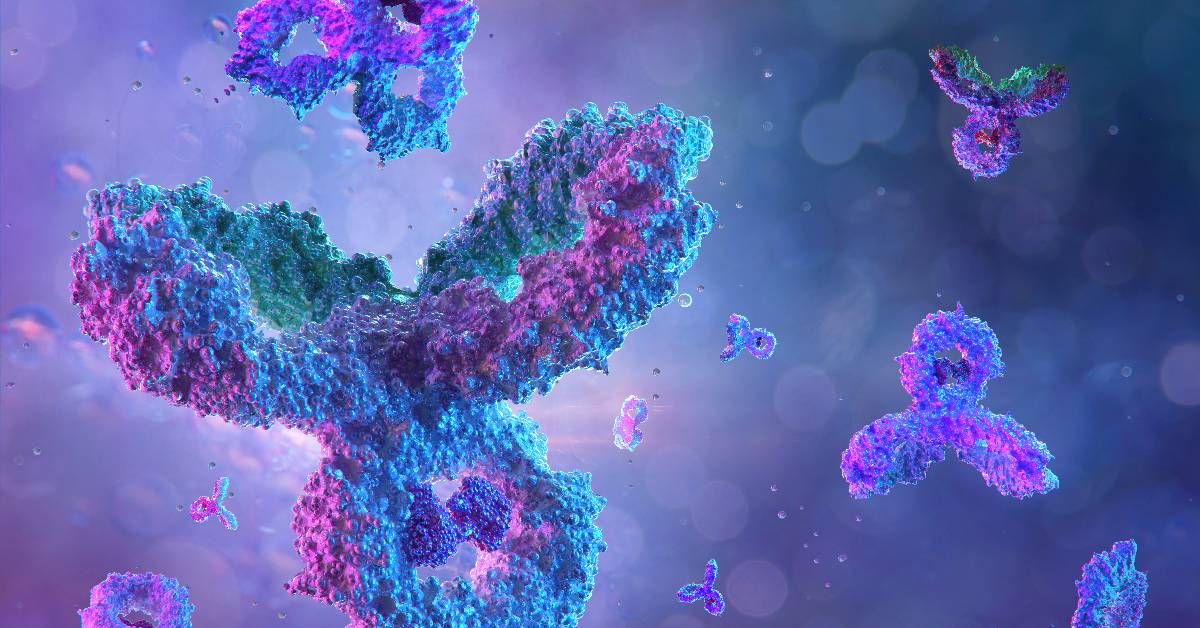
Big data has been a big thing for most of the last decade, yet its importance in industry continues to increase at a rapid rate. This is being driven both by the amount of data available and the increased processing power available to handle that data. Open AI’s GPT-4 is, of course, the latest example of what can be achieved when you harness huge quantities of data. This enormous potential means that big data has made its way into almost every industrial setting – the maritime sector is no exception.
In fact, the use of big data to improve operations will be critical in maritime transport. This is especially the case for shipping, in which the current climate includes overcapacity, a shortage of labour, and the recent introduction of new regulations (EEXI and CII) concerned with meeting minimum environmental thresholds. Big data’s importance is not limited to the big ships though. For example, the rapid growth of offshore wind and other marine energy technologies will demand large fleets of vessels servicing those projects. As those fleets grow there will be an increased need to manage them in a data driven manner.
One focus is the management of fuel consumption. Fuel represents a major cost component in operating a vessel. In shipping, for example, fuel costs can make up to half of total operating costs. Fuel consumption also has a direct effect on the emissions of a vessel, and therefore on a vessel’s impact on the environment. Traditional systems can measure a vessel’s historical fuel efficiency, but to minimise fuel consumption it can be useful to make predictions about fuel efficiency for future trips. That is not a simple proposition – the fuel efficiency of a vessel is dependent on an array of interrelated variables, including the weather, current, wave height and direction, engine type, vessel characteristics, etc. This can make it difficult to determine how to operate a vessel to maximise fuel efficiency using traditional methods. So, the industry has looked to big data and machine learning models, which are particularly effective at making predictions based on complex sets of interrelated variables.
One way is to use it to control the vessel. We’ve previously discussed, for example, how autonomous technologies could improve efficiency in the future. For now though, the most practical solutions give insight to crewmembers who can use that insight to inform decisions on how best to operate a vessel. For example, Stena (one of the world’s largest ferry operators) expects to save 5% in fuel usage by implementation of the “Stena Fuel Pilot”, which uses AI to assist the captain and crew in operating a ferry to minimise fuel consumption.
Another example is Shell’s patent-pending JAWS application (developed in collaboration with the University of Southampton), which instructs an operator on the optimum trim (forward/backward tilt) and draft (amount of a vessel below the waterline) based on in-service conditions such as weather and hull fouling. As trim and draft change due to the consumption of fuel carried by the vessel, JAWS can instruct an operator on how to adjust trim, draft and speed to maintain maximum fuel efficiency.
Big data is not only useful once a vessel has left port though. Route planning plays an enormous role in ensuring fleets are operated as efficiently as possible. One example is DeepSea’s product, Pythia. This aims to improve on weather routing (providing an optimized route based on forecasted weather) by considering a detailed understanding of the vessel and how it responds to various conditions. By doing this, DeepSea says Pythia can achieve up to 12% in fuel savings. It is these types of savings that have no doubt motivated BAS AI labs to develop its own system for recommending the most fuel-efficient route in polar conditions, where sea ice must be navigated. This system will be used by RRS Sir David Attenborough (almost Boaty McBoatface) to navigate polar regions while minimising its impact on the environment.
Clearly, fuel efficiency is important. There are, however, other areas of operating a vessel where big data has found use. A big topic, for example, is safety of crew and passengers. Orca AI addresses this with a collision avoidance platform that detects ships, ranks them according to collision potential, and alerts crew when there is any potential for contact. Ørsted, on the other hand, is particularly concerned about movement of personnel between a vessel and an offshore structure such as a wind turbine. It has filed a patent application for a system that predicts (based on wave detection) whether movement of the bow of a vessel will be acceptable for transfer of a person from the vessel.
Both safety and fuel efficiency of a vessel are somewhat dependent on the vessel operating as it is expected to do so. This means maintaining or replacing components before they fail. Traditionally, vessels are inspected and maintained on a schedule. Predictive maintenance instead involves real-time monitoring of components to estimate when maintenance should occur. The sheer number of components that make up a vessel, and the seemingly unpredictable impact of the harsh ocean environment, make this a particularly difficult task. This is, of course, where big data comes in. We have already explained that big data and machine learning algorithms are particularly good at solving this type of problem. Wärtsilä’s Expert Insight product is a great example of this – it identifies anomalies in engine performance by comparing values measured by sensors in the engine with those produced by an AI-based model of how the engine should be operating.
This, like other examples above, can provide an operator with significant cost savings. And this is a big reason why big data is really taking off in the maritime space – it has the potential to completely transform the economics of operating a vessel. We think this, and the potential for significant improvements in sustainability of maritime transport, make big data in the maritime sector a really interesting space to watch.
Ben is a Senior Associate and Patent Attorney at Mewburn Ellis. He is experienced in patent drafting, prosecution and Freedom to Operate within the mechanical engineering, medical device and consumer products sectors. Ben also deals with filing and infringement issues relating to registered and unregistered designs. He has a Master’s degree in Intellectual Property Law from the Univeristy of Melbourne. He also holds a Bachelor of Engineering (Mechanical) and Bachelor of Commerce (Finance) from the University of Queensland.
Email: ben.boyd@mewburn.com
Our IP specialists work at all stage of the IP life cycle and provide strategic advice about patent, trade mark and registered designs, as well as any IP-related disputes and legal and commercial requirements.
Our peopleWe have an easily-accessible office in central London, as well as a number of regional offices throughout the UK and an office in Munich, Germany. We’d love to hear from you, so please get in touch.
Get in touch