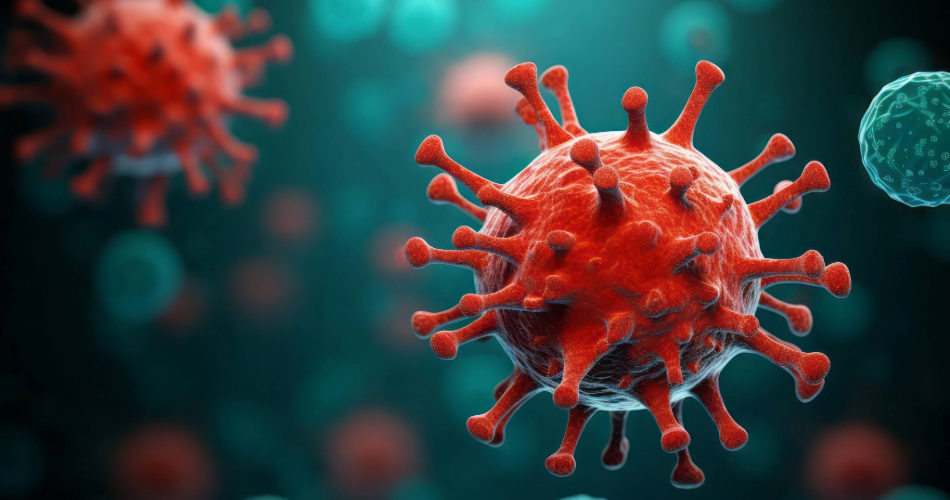
The human microbiome is very diverse. A typical gut microbiome can contain hundreds or even thousands of unique bacterial species. Disruptions to the microbiome can affect many aspects of human health and disease. For example, dysbiosis (an imbalance of the species within the microbiome, often involving a reduction in microbiome diversity) has been linked with a number of conditions including gut disorders like inflammatory bowel disease (IBD), obesity, malnutrition, and even cancer. Understanding the interactions between species and the number of different species present is therefore important in our attempts to address such conditions.
However, quantifying the diversity of the human microbiome is challenging. It traditionally involves culturing a microbiome sample, and then trying to manually select different bacterial colonies for isolation. However, this is a laborious and time intensive process. It is also difficult to replicate, as it is rare for the picker to be able to quantify their picking criteria (think of a researcher having to note down each colony’s density, size and colour as they pick them).
These challenges are being addressed by a new research tool – CAMII. CAMII is a bacterial colony picking robot (CAMII stands for Culturomics by Automated Microbiome Imaging and Isolation) that can greatly increase the number of unique bacterial species cultured from human microbiome samples. The robot can detect differences in morphology between bacterial colonies (such as colony density, size and colour). CAMII also takes over the labour of colony picking, so it can then pick a diverse group of colonies for culturing.
The researchers behind CAMII published a paper in Nature, describing CAMII and how its image guided “smart-picking” strategy was able to isolate a substantially more diverse set of species as compared to random isolation. Putting CAMII to work on microbiome samples from 20 individuals resulted in the creation of highly diverse biobanks containing 394 unique 16S ASVs, which included more than 80% of the ASVs by abundance that were identified in the original samples.
The researchers were also able to use the conservation of morphological characteristics within specific bacterial genera to train a machine learning model that allowed CAMII to identify specific bacteria from their morphology alone.
CAMII has an imaging platform that allows it to photograph colonies and extract morphological information. The pipeline starts with a microbiome sample. This sample is processed to isolate the gut microbiota and then dispensed onto agar plates to allow the various bacteria in the sample to propagate and form colonies.
CAMII begins by taking a raw photo of the agar plate and then tries to detect the “edge” of each bacterial colony. Essentially, CAMII detects pixels that have a highly contrasting colour value to a neighbouring pixel. Hopefully this corresponds to the edge of a bacterial colony which contrasts with the blank background of the image. From here, CAMII identifies how these points join up to find the perimeter of each bacterial colony.
CAMII then needs to distinguish between a real colony, and other features on the agar plate such as debris or dead cells. It does this by relying on the typical morphological features of bacterial colonies, such as their typical size and generally circular appearance.
CAMII filters the colonies it has identified based on several features, including size, circularity and convexity. Certain colonies then enter post-processing, which helps to resolve the edge cases (i.e. where a colony has been determined to have a “poor” circularity – this may be because multiple colonies overlap, affecting the shape of the perceived colony).
After all the colonies have been identified, CAMII uses its “smart-picking” algorithm to maximise the morphological diversity of the colonies it picks for isolation and identification. This strategy significantly out-performs a random picking approach, creating highly diverse sample sets much faster. It is also much more consistent than a human picker, enabling more reliable studies in the future.
As the researchers who created CAMII had found that bacterial colonies of different genera had different morphological characteristics, they decided to explore whether CAMII would be capable of picking specific types of bacteria based on their colony morphology alone. This could save researchers a lot of time when seeking to study a particular bacterial species.
The researchers trained a classification model using the morphology and taxonomy data from random colonies that made up 70% of the identified isolates in a dataset. This machine learning model was then tested on the remaining 30% of isolates and achieved around a 70% prediction accuracy for genera with over 100 isolates within the training dataset. This is a remarkable level of precision when using morphological data alone, and researchers believe the accuracy can be improved by using more sophisticated machine learning models or increasing the morphological data collected.
Interestingly, the researchers found that colony morphology was more highly conserved within isolates taken from the same person but was more variable between isolates taken from different people. This indicates that even within the same species of bacteria, we are carrying unique strains with differing morphology.
CAMII shows the possibilities that automation and machine learning have at moving the microbiome space forward. It is a tool with great promise to help researchers continue to make advances in this exciting field.
Isobel is a trainee patent attorney in the life sciences team. She has a BSc in Biochemistry from Imperial College London. Her final year project involved comparing the genomic response of human and rats post burn to help understand the evolution of the burn response in humans.
Email: isobel.fisher@mewburn.com
Our IP specialists work at all stage of the IP life cycle and provide strategic advice about patent, trade mark and registered designs, as well as any IP-related disputes and legal and commercial requirements.
Our peopleWe have an easily-accessible office in central London, as well as a number of regional offices throughout the UK and an office in Munich, Germany. We’d love to hear from you, so please get in touch.
Get in touch