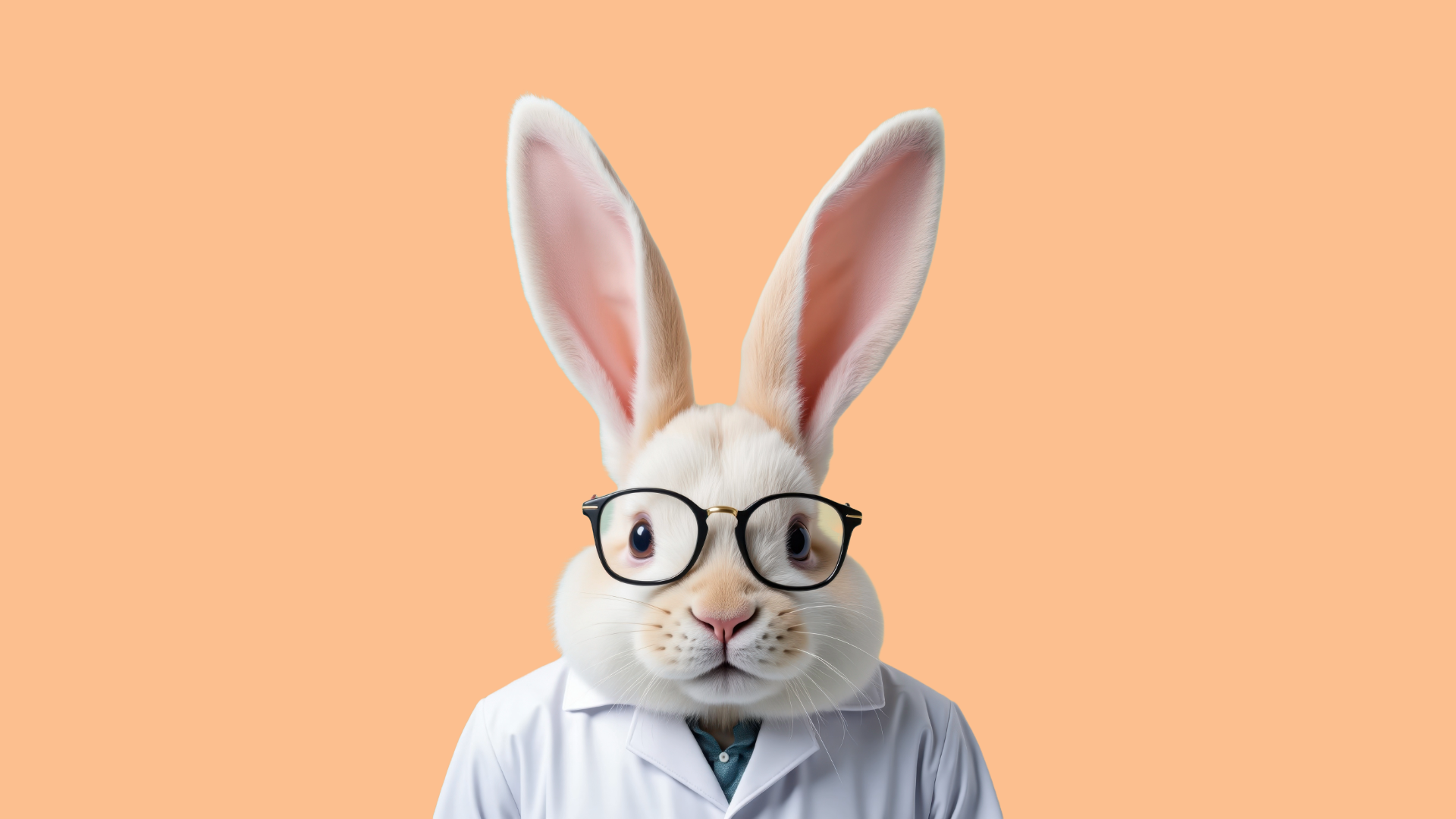
For those of us who studied Biochemistry at college, the classic active site “catalytic triad” of serine peptidases was often our first introduction to the fascinating world of enzymes (and also, happily, something of a ‘banker’ question in exams).
The triad itself is composed of the amino acid serine (giving the enzyme class its name) as well as histidine and aspartic acid. These residues work together dynamically in several steps to split substrates such as proteins by a mechanism based on ‘nucleophilic attack’. In the natural world the basic spatial arrangement of this active site is well conserved but different enzymes have different backbones or frameworks to provide it, leading to different properties.
Last month a couple of reports, within a day or two of each other, caught my eye: both concerning these enzymes in quite different ways.
The first was a report that researchers from David Baker’s lab in Seattle utilised a novel computational method for designing entirely de novo serine hydrolase enzymes that could efficiently catalyse hydrolysis of several model esters.
The second was that Dave Estell, a fellow and laureate at our client International Flavors & Fragrances Inc., has been elected as a member of the National Academy of Engineering for his long standing role in the development of protein engineering technologies.
Dave is an inventor on many patents relating to serine peptidases, and we have been fortunate to work with him and his co-inventors for many years on these technologies. The protein engineering which underlies those patents typically involved laborious side-directed mutagenesis of individual amino acids to improve the enzymes and adapt them for industrial use. Such patents have been the subject of many EPO oppositions and Board of Appeal decisions, an early example of which is T915/94 concerning an application first-filed more than 40 years ago. In upholding the patent the Board summarised the challenge of protein engineering at that time:
“The technical problem to be solved on the basis of this teaching is to apply enzyme engineering to subtilisins, a technique which is possible only if one first identifies correct sites for mutation….it is worth remarking that the fact that finding "respective positions" was within the reach of the skilled person…does not render obvious the problem of "identifying correct sites for mutation among the about 275 sites of the mature protein". This is because finding "respective positions" does not automatically imply that these "respective positions" are good for the purpose of substituting and obtaining variation in properties of the enzyme in ways that are very likely to be useful in terms of certain physical features (eg, pH activity, oxidation stability), while still retaining enzyme function.”
Fast forward to 2025 and the Baker group’s publication in Science introduces a framework relying on AI tools for creating novel serine hydrolase enzymes capable of catalyzing complex, multistep reactions just like their natural counterparts.
The authors set out to model how to design an enzyme that could stabilize key states along the serine hydrolase reaction pathway using a deep neural network called PLACER (Protein-Ligand Atomistic Conformational Ensemble Resolver) which had been trained on the structures of protein-small molecule complexes in the Protein Data Bank.
To design novel enzymes with complex catalytic sites the team used the established generative capabilities of RFdiffusion along with PLACER to predict the conformations of the active site in each step of the chemical reaction, seeking to ensure the catalytic sidechains adopted the active conformation throughout the reaction mechanism.
The approach eventually resulted in the team generating enzymes with a minimal active site specification while maintaining high catalytic efficiency, particularly with certain model substrates. These novel enzymes contained structures that closely matched the computational design models, as confirmed by crystal structure analysis. However they had novel backbones, with some folds not found at all in natural serine hydrolases.
Certainly quite a paradigm shift from the approach of painstakingly identifying potentially useful target positions discussed by the Board of Appeal in their decision a few decades earlier!
As we have reported, AI has been used to design enzymes before. However in designing enzymes de novo using the PLACER and RFdiffusion tools the Baker lab have provided a roadmap for designing entirely novel enzymes that catalyse multistep transformations and which might eventually address some of the key industrial and pharmaceutical problems of our age such as degrading plastics or efficiently providing new chemical feedstocks.
Interestingly the report notes that a provisional patent covering the PLACER network has been filed and licensed to the early-stage biotechnology company Vilya. While the technology has certainly moved on, its notable that the importance of patent protection remains!
Simon has more than 25 years’ experience working as a European and UK patent attorney working in the life sciences sector. He provides clear and practical advice on all aspects of patents and other IP rights including drafting, prosecution, inter partes proceedings and due diligence. He is regularly involved in oppositions and appeals at the European Patent Office and covers a wide variety of technical fields, including physical biochemistry, therapeutics, diagnostics, genetics, chemistry and mechanical subject matter.
Email: simon.kremer@mewburn.com
Our IP specialists work at all stage of the IP life cycle and provide strategic advice about patent, trade mark and registered designs, as well as any IP-related disputes and legal and commercial requirements.
Our peopleWe have an easily-accessible office in central London, as well as a number of regional offices throughout the UK and an office in Munich, Germany. We’d love to hear from you, so please get in touch.
Get in touch